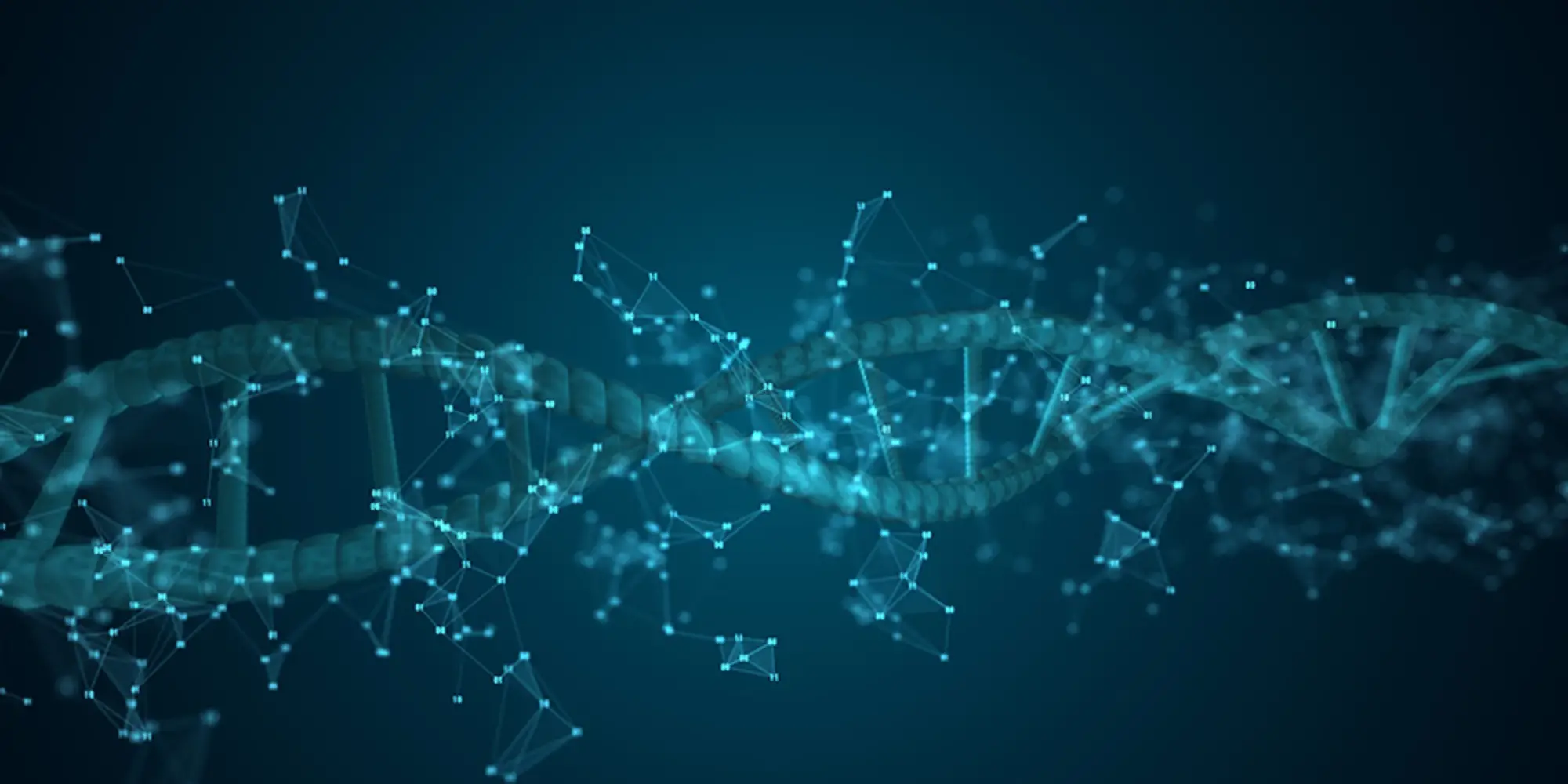
Using AI To Predict Gene Expression: New Foundational Model Could Improve Understanding of Biology
Media Inquiries
Computer simulation has long been a first step in the manufacturing process. Before an engineer or scientist working in fields like energy, chemistry or chip design makes a product or carries out an experiment, they routinely run a simulation.
But biology has yet to tap into the full benefits of computer simulations. Biologists still use lab experiments to test most hypotheses, including, for example, how cell activities change when one gene is turned off or perturbed.
A new artificial intelligence foundation model called general expression transformer(opens in new window) (GET), developed by an international group of scientists including Carnegie Mellon University's Eric Xing(opens in new window), seeks to change this process. GET simulates how genes are turned off and on in different biological scenarios, also known as gene expression. Researchers describe GET in a study recently published in Nature(opens in new window), and note that it could make biological experiments faster and more affordable to run.
"Biology is the last science still relying on the physical lab as the initial step," said Xing, a professor in CMU's School of Computer Science(opens in new window). "Our vision is that we use the AI foundation model to try different hypotheses, to predict and simulate, and the wet lab becomes the last step of validating the highly plausible hypotheses."
GET was built from both sequencing data and DNA accessibility data. Sequencing data refers to the specific order of the four nucleotide bases — adenine, thymine, cytosine and guanine — that make up DNA. This sequence is the genetic code that cells use to grow and operate.
Some sections of DNA also contain information that governs how the DNA sequences act. This information is provided in part through DNA accessibility data, which includes how accessible certain gene sequences are and how various molecules operate and behave inside the cell. For example, DNA in one region could form a gene that contains information about where a specific protein should dock. DNA in another region could contain information about how likely a section of the chromosome will be to open so enzymes can bind to it.
"This is called the activity information, which usually is the secondary information in DNA," Xing said.
Gene activity allows scientists to understand how different parts of the cell work together to support its normal function, and how that workflow is affected by changes in the environment, such as a viral infection. Given a sequence and its accessibility, GET can predict the gene's activity in terms of where and when it expresses under disease or normal conditions.
"Current language models and other foundation models don't yet excel at understanding the activity aspect of the genes," said Xing. "They are good at interpreting the coding sequences that program the structure of molecules like proteins and determining their function, but not their activity — when and where the gene needs to be active or shut down."
Factoring in gene activity is important. Whether a cell's health is negatively affected by disease cannot be determined just by looking at it or reading its sequence. But particular patterns in gene expression activity could provide a hint, such as a set of genes in one location shutting down when they are normally on. GET could highlight possible anomalies in this area. In the paper, for example, Xing and his colleagues used the model to determine the specific subtypes of leukemia cells.
GET has been trained on more than 200 cell types, which researchers hope to expand on and diversify in a future phase. At last month's Conference on Neural Information Processing Systems(opens in new window), Xing and his collaborators soft-launched the next generation of models for DNA, RNA, protein and single-cell level activities, along with their startup, GenBio.ai(opens in new window), under a holistic vision called AI-Driven Digital Organism(opens in new window) (AIDO) — a multiscale foundation model system that can predict, simulate and program biology at all levels.
"AIDO models can also be used in the future to determine the impact of treatment and drugs," Xing said. "We want all these models to talk to each other so you can start to answer biological queries more holistically. All this makes the problem more complex but more realistic."
To design protein structures, scientists will ultimately need to determine the DNA sequence, as well as promoters and regulators that control the protein's activity. Xing's work focuses on connecting the links between all the different factors that go into making and managing proteins.
"Proteins have to exist in a very rich and messy context," Xing said. "Current modality-specific models do not give you enough information to determine the final effect of a designed protein. It gives you some kind of starting point for you to test in a wet lab. We want to go further to allow the entire cellular environment to be simulated."