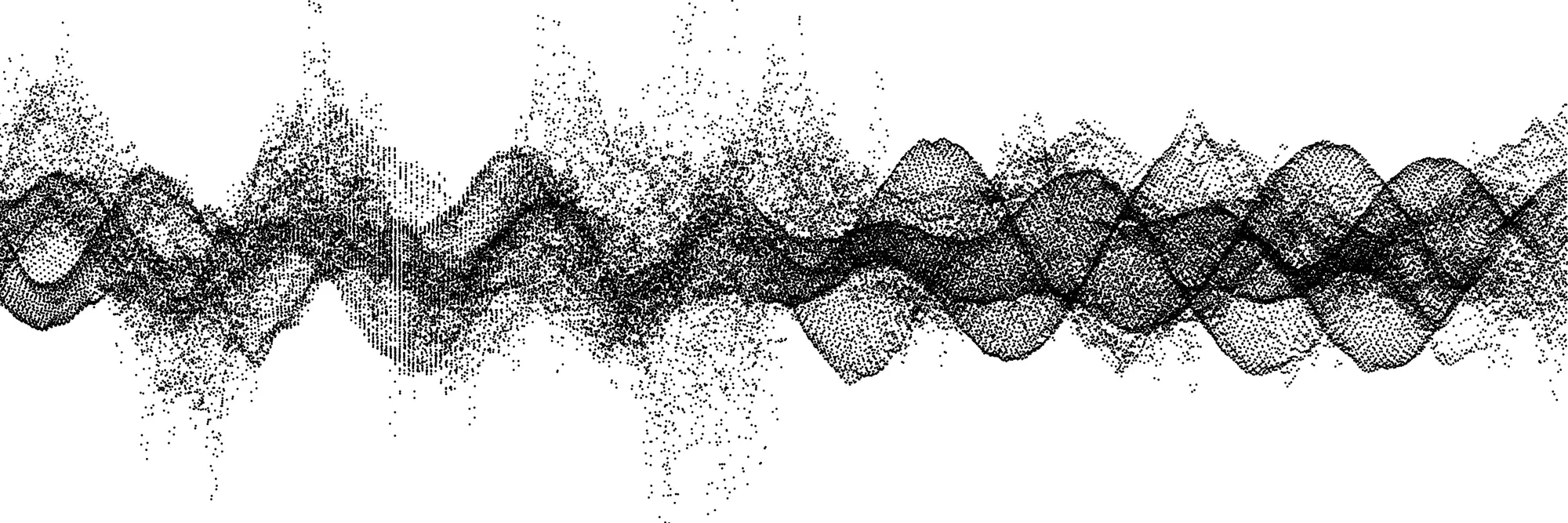
Distinguishing Real Sounds from Deepfakes
Media Inquiries
Deepfake videos generated by artificial intelligence grow increasingly difficult to identify as false, a challenge that could significantly skew the results of the upcoming presidential election.
Laurie Heller(opens in new window), Carnegie Mellon University professor of psychology, collaborated with Hafsa Ouajdi, Oussama Hadder, Modan Tailleur and Mathieu Lagrange of École Centrale Nantes(opens in new window) to analyze the errors made by the first deep neural network detector the research team developed to automatically classify environmental sounds as either real or AI-generated.
The research team published their findings in the paper "Detection of Deepfake Environmental Audio,"(opens in new window) which they presented on Aug. 27 at the 32nd European Signal Processing Conference (EUSIPCO 2024)(opens in new window) in Lyon, France.
Environmental sounds are defined as the background noise of a recording — any sound excluding speech and music. These sounds could include such things as a car driving by or a door closing in another room.
The detector that the research team developed is currently limited to identifying seven categories of environmental sounds. In testing the environmental sound detector, the École Centrale Nantes team found it to be incredibly accurate, ultimately resulting in about 100 errors out of about 6,000 sounds.
Analysis revealed the two types of errors the detector could make. The detector could either label an AI-generated sound as real, or a real sound as AI-generated. Heller’s study aimed to determine whether a human could find audible clues that the detector missed, causing them to judge some of the missed real sounds as real, or some of the missed AI-generated sounds as fake.
Heller’s study consisted of 20 human participants, who listened to the same sets of sounds that the detector identified incorrectly. Like the detector, participants were tasked with identifying which of the sounds they heard were real and which were AI-generated. The real environmental sounds used in the study were sourced from publicly available databases. The AI-generated environmental sounds were taken from the winners of a competition in which applicants submitted sounds developed using AI, with the winning sounds being the most accurate or real.
For fake sounds that the detector judged were real the results of the human study were inconclusive. Humans were accurate about 50% of the time, indicating that they were not sensitive to the fakeness of sounds which fooled the detector. Participants might have not been able to definitively classify the sounds they were hearing, with the results reflecting chance choices rather than reliable answers.
However, for the real sounds that the detector judged were fake, humans were correct around 71% of the time and were more accurate than the detector. This statistic reveals that the answers were not a result of chance, but rather the participant’s definitive and correct classification of the real sounds. Heller concludes that these results imply that there might be some sort of cue in these real environmental sounds that humans are able to detect, but that the detector fails to recognize. If researchers can identify this hypothesized cue, the AI sound detectors could be improved to increase their accuracy.
The environmental sound detector and Heller’s results can lead to the development of more complex AI detection tools. Prior AI sound detectors were made to only identify speech, but with the environmental sound detector, researchers eventually could reach a point in which detectors can analyze more complex recordings of both speech and environmental sounds.
Further research to improve AI detection tools is crucial in keeping up with AI-driven deepfake technologies that are quickly advancing in their capabilities.
“We’re at a point where the public is going to underestimate that ability, and it’s rapidly getting better,” Heller said. “A worst-case scenario would be to end up in a society where AI is so advanced that humans aren’t able to tell what is real or what is artificial. We want to be prepared before that happens.”
Heller also mentioned the importance of implementing policies that can regulate AI-driven media components.
“Everything generated using AI should have a flag on it,” she suggested.