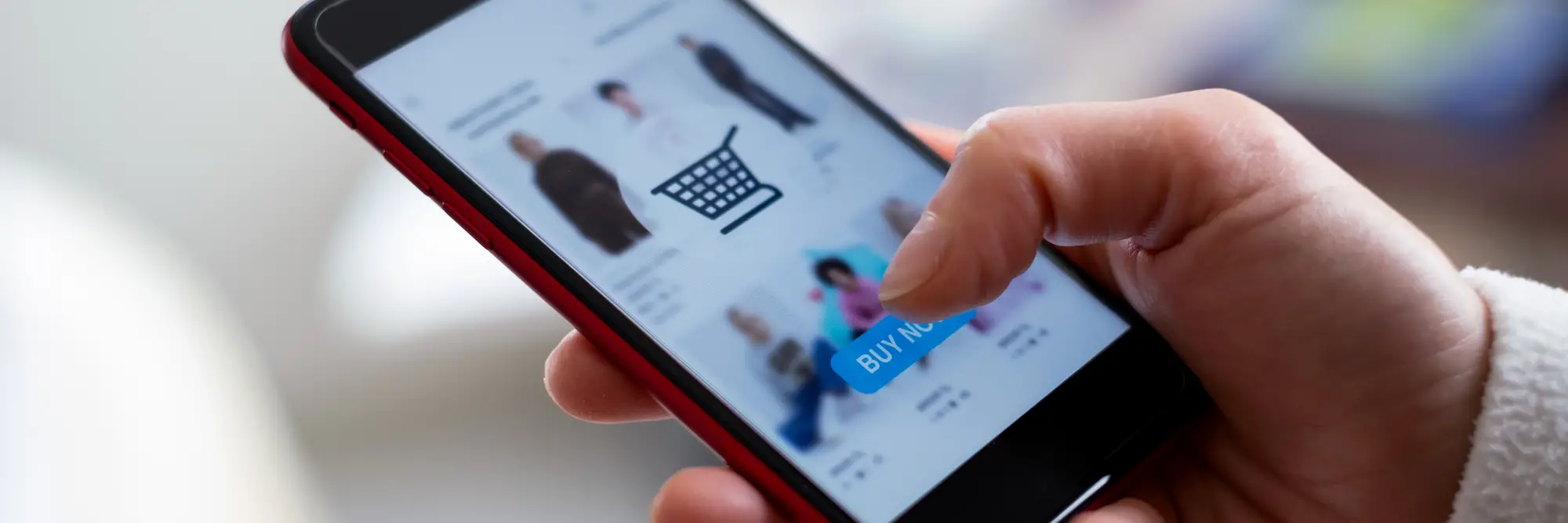
Tepper School Study: Enhancing Online Retail Sales Forecasting Using Clickstream Data
Media Inquiries
In a new study, researchers developed a model that helps online retailers to more accurately predict customer purchases from a wide range of products in complex online shopping environments, reducing sales forecast errors by 10%–30% compared to traditional methods. Their study, titled "A High-Dimensional Choice Model for Online Retailing(opens in new window)," is published in the journal Management Science.
Researchers from Carnegie Mellon University, the University of Michigan, and Washington University propose this method to tackle challenges brought by digital advancements in the retailer space. While online shopping offers greater convenience to consumers, it becomes more complex for retailers to manage. Significant efforts are required for effective pricing, inventory, and assortment decisions, as online retailers often offer a variety of product choices that outstrip those of their brick-and-mortar counterparts.
"This work offers online retailers a useful tool to improve their operational decisions in this challenging new online retail environment," said Zhaohui (Zoey) Jiang(opens in new window), study co-author and assistant professor of business technologies at Carnegie Mellon’s Tepper School of Business(opens in new window). "Our model's ability to flexibly and accurately understand substitution patterns among a large number of products, without sacrificing scalability, lays an important foundation for effective operational decision-making."
Traditional methods often struggle in settings with a large number of products due to their limited ability to accurately track how consumers switch across products, restricted by a narrow set of observable and quantifiable product features as well as scalability challenges. While large tech firms like Amazon have the computational power and tracking technologies, their proprietary methods are not accessible to smaller retailers.
By using consumer clickstream data and combining econometric with machine learning techniques, the researchers created a computationally simple model that not only provides a flexible and accurate understanding of product substitution patterns, but more importantly, scales up easily as retailers' assortments grow. These patterns inform retailers how changes in one product (e.g., price or availability) affect the sales of others, which is a crucial input for many decisions such as assortment planning, inventory management, and pricing strategies.
Jiang and her co-authors demonstrate this new method offers more accurate demand forecasts in a wide range of scenarios compared with traditional models, reducing prediction error by 10%–30%. More importantly, the method does not require highly extensive computational power and excels in precisely recovering substitution patterns across products, reducing the percentage deviation from the true values by approximately half.
The authors suggest that this method can be readily applied by both large and small online retailers managing complex product choice sets across various business settings. This includes demand estimation for inventory and transshipment decisions (moving goods from one vessel to another), as well as a variety of other operational decision areas such as promotion and assortment. However, Jiang also noted that more research is needed to see how this method can be adapted for new products and in settings where online clicks aren't available, like in physical stores.
Additional authors of the study include Jun Li from the Ross School of Business at the University of Michigan, and Dennis Zhang from the Olin Business School at Washington University in St. Louis.