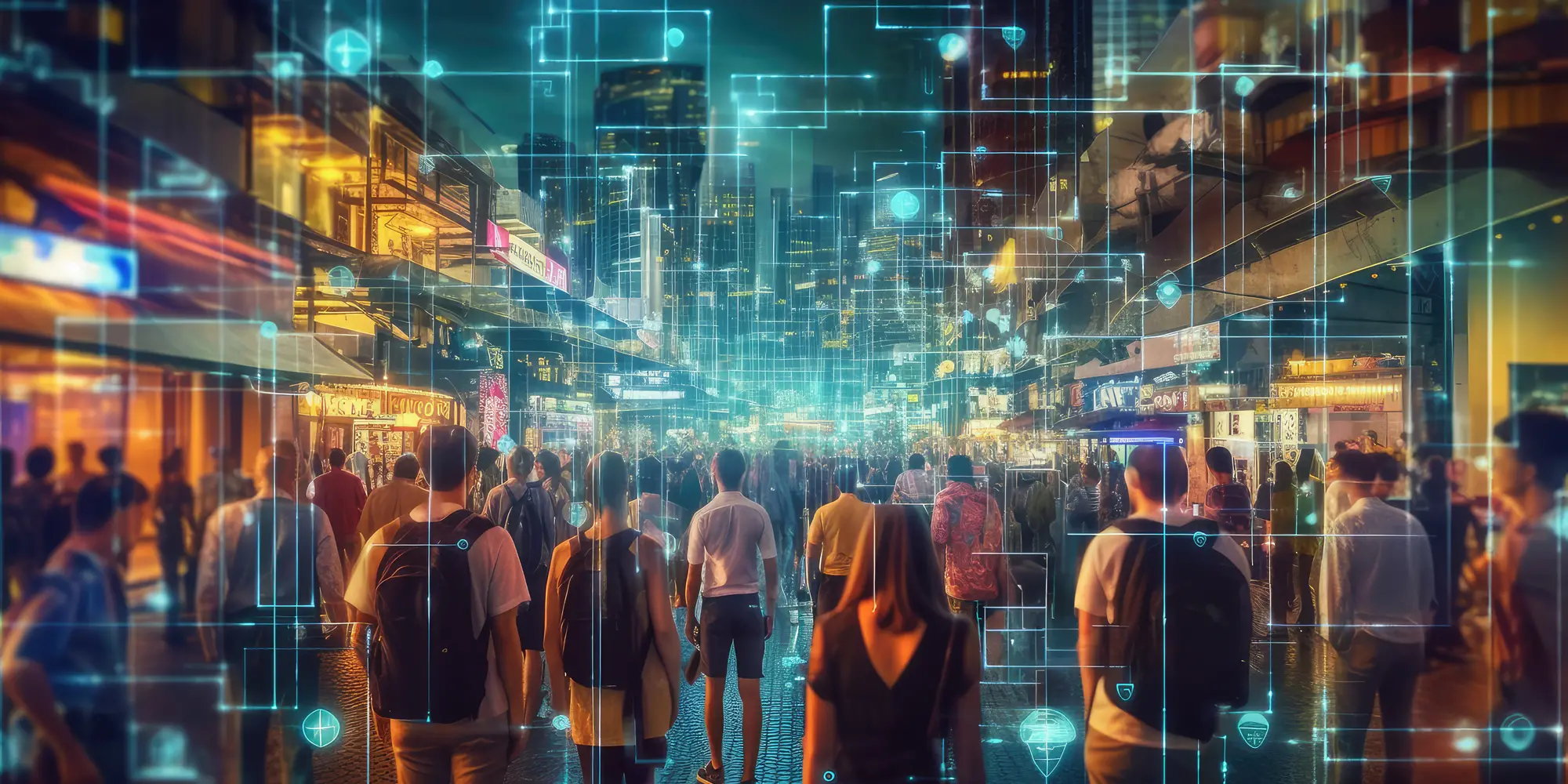
Addressing Copyright, Compensation Issues in Generative AI
New Carnegie Mellon Research Tackles Challenges in Emerging AI Tools
Media Inquiries
Recent work by Carnegie Mellon University researchers tackles the thorny issues of copyright and compensation for generative AI models that create new images.
A team in the School of Computer Science's Generative Intelligence Lab collaborated with Adobe Research and the University of California, Berkeley, to develop two algorithms to help generative AI models take important steps on these issues. The first algorithm prevents these models from generating copyrighted materials, while the second develops a way to compensate human creators when models use their work to generate an image.
Image-generating models such as DALL-E 2, Midjourney and Stable Diffusion are powerful tools for creating realistic visual content from a simple text description. Behind the scenes, these models were trained on millions to billions of internet images, some of which might be copyrighted material, licensed images and personal photos.
"As researchers in this field, we are responsible for addressing the social issues that come with it," said Jun-Yan Zhu(opens in new window), an assistant professor in the Robotics Institute(opens in new window) and head of the Generative Intelligence Lab, which is working to address the ethical and social issues related to generative AI. "Creating technologies to address these issues is only one aspect. We also need more work in both legislation and how to regulate AI."
The research teams will present two papers at the International Conference on Computer Vision 2023(opens in new window) this October.
The first paper, "Ablating Concepts in Text-to-Image Diffusion Models(opens in new window)," helps AI generative models avoid creating specific copyrighted images or styles.
For example, if you ask an AI program for a painting by a living artist, it will generate an image that closely resembles that artist's style. The algorithm the CMU researchers propose aims to prevent this and instead makes the AI model generate a generic painting.
"We can use this as an option when an artist wants to opt out of an AI model at any point in time," said Nupur Kumari, a Ph.D. student in robotics and the paper's lead author. "It creates more control and freedom for people and companies who don't want their images to be used."
The second paper, "Evaluating Data Attribution for Text-to-Image Models(opens in new window)," develops a method for compensating people and companies whose data is used to train the AI. The algorithm attempts to determine how much each training image contributes to a generated image. It could be used to fairly distribute payments to the owners of copyrighted images in AI databases.
If you ask an AI model to generate an image of a watercolor painting, for example, the resulting image will be influenced by some artists who work in watercolors. This new algorithm aims to quantify how much each artist contributed to this new piece of synthetic artwork.
"We're working to answer the question, 'Which set of images influenced the synthesized image?'" said Sheng-Yu Wang, a Ph.D. student in robotics and the paper's lead author. "We can potentially use this algorithm to assign credits to data contributors. Eventually, the goal is to fairly compensate data owners who contribute to the creation of generative AIs."
The new algorithms are still in the early stages of development, and the authors admit many questions remain unanswered. It's unclear whether the copyrighted content has been completely removed or just hidden somewhere, for example, and more study is needed to explain how an attribution algorithm assesses each training image's influence.
Despite the unanswered questions, the new algorithms pave the way for addressing copyright issues across generative AI platforms and take the first steps toward compensating people and companies whose work contributes to AI images.