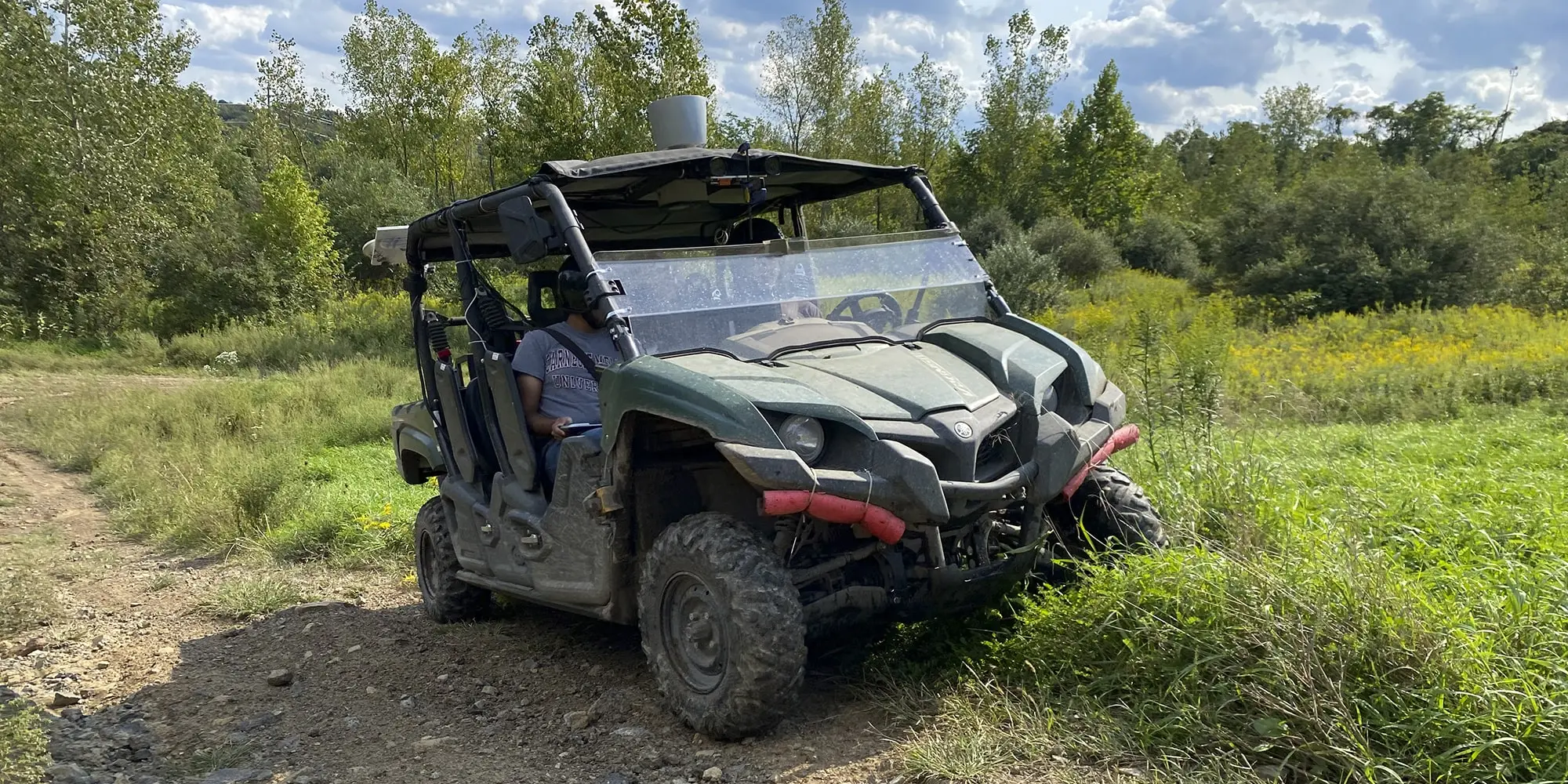
Roboticists Go Off Road To Compile Data That Could Train Self-Driving ATVs
TartanDrive dataset likely largest for off-road environments
Media Inquiries
Researchers from Carnegie Mellon University took an all-terrain vehicle on wild rides through tall grass, loose gravel and mud to gather data about how the ATV interacted with a challenging off-road environment.
They drove the heavily instrumented ATV aggressively at speeds up to 30 miles per hour. They slid through turns, took it up and down hills, and even got it stuck in the mud — all while gathering data such as video, the speed of each wheel and the amount of suspension shock travel from seven types of sensors.
The resulting dataset, called TartanDrive(opens in new window), includes about 200,000 of these interactions. The researchers believe the data is the largest real-world, multimodal, off-road driving dataset, both in terms of the number of interactions and types of sensors. The five hours of data could be useful for training a self-driving vehicle to navigate off road.
"Unlike autonomous street driving, off-road driving is more challenging because you have to understand the dynamics of the terrain in order to drive safely and to drive faster," said Wenshan Wang(opens in new window), a project scientist in the Robotics Institute(opens in new window) (RI).
Previous work on off-road driving has often involved annotated maps, which provide labels such as mud, grass, vegetation or water to help the robot understand the terrain. But that sort of information isn't often available and, even when it is, might not be useful. A map area labeled as mud, for example, may or may not be drivable. Robots that understand dynamics can reason about the physical world.
The research team found that the multimodal sensor data they gathered for TartanDrive enabled them to build prediction models superior to those developed with simpler, nondynamic data. Driving aggressively also pushed the ATV into a performance realm where an understanding of dynamics became essential, said Samuel Triest, a second-year master's student in robotics.
"The dynamics of these systems tend to get more challenging as you add more speed," said Triest, who was lead author on the team's resulting paper. "You drive faster, you bounce off more stuff. A lot of the data we were interested in gathering was this more aggressive driving, more challenging slopes and thicker vegetation because that's where some of the simpler rules start breaking down."
Though most work on self-driving vehicles focuses on street driving, the first applications likely will be off road in controlled access areas, where the risk of collisions with people or other vehicles is limited. The team's tests were performed at a site near Pittsburgh that CMU's National Robotics Engineering Center(opens in new window) uses to test autonomous off-road vehicles. Humans drove the ATV, though they used a drive-by-wire system to control steering and speed.
"We were forcing the human to go through the same control interface as the robot would," Wang said. "In that way, the actions the human takes can be used directly as input for how the robot should act."
Triest presented the TartanDrive study at the International Conference on Robotics and Automation(opens in new window) (ICRA) in Philadelphia. In addition to Triest and Wang, the research team included Sebastian Scherer(opens in new window), associate research professor in the RI; Aaron Johnson(opens in new window), an assistant professor of mechanical engineering; Sean J. Wang, a Ph.D. student in mechanical engineering(opens in new window); and Matthew Sivaprakasam, a computer engineering student at the University of Pittsburgh. Their paper has been nominated for ICRA's Outstanding Learning Paper.